Adverse Drug Event Detection Using Spark NLP
Adverse drug events (ADEs) are a significant cause of morbidity and mortality. In real-world clinical practice, ADEs are often reported spontaneously without formal coding or structured documentation in patients’ electronic health records. Natural language processing (NLP) of clinical text is a potential mechanism for identifying ADEs with better efficiency and accuracy.
In this talk, we will share our experiences in working with Spark NLP for Healthcare models and pipelines for detecting ADEs present in unstructured clinical data.
We will discuss ADE identification for specific medications in a variety of clinical scenarios and provide examples of how NLP of clinical text can provide a deeper insight into the real-world patient experience.
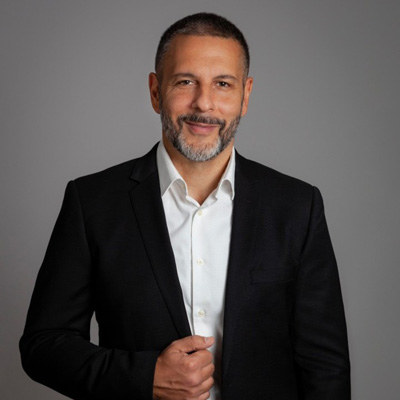
Lawrence Rasouliyan
Head of Biostatistics and Data Science at OMNY Health
Lawrence Rasouliyan is Head of Biostatistics and Data Science at OMNY Health. He has over 20 years of experience in the design, execution, analysis, and reporting of clinical and observational studies to support real-world evidence generation. Lawrence has worked extensively as a data scientist and biostatistician in the health research industry while collaborating with a wide range of practice areas including epidemiology, health economics, patient-centered outcomes assessment, market access strategy, and medical affairs. He has a deep interest in applying advanced analytics to real-world topics to help improve patient conditions and experiences.
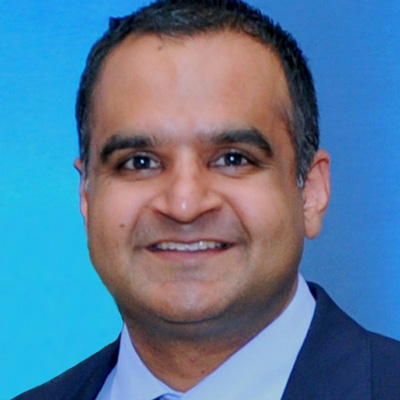
Vikas Kumar
Senior Data Scientist at OMNY Health
Vikas Kumar is a Senior Data Scientist at OMNY Health. He is an MD-turned-data-scientist with an interest in improving healthcare via computing and data science techniques. Over the past few years, Vikas has authored a book on healthcare analytics and contributed to two online healthcare informatics courses. He has also contributed to several poster presentations that were accepted into leading healthcare conferences on the topics of real-world clinical evidence and natural language processing.