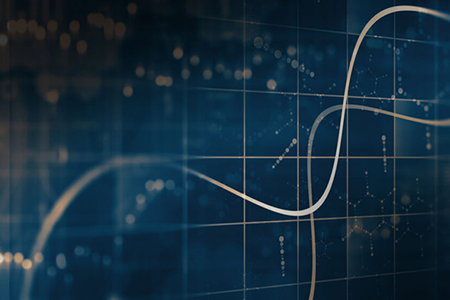
Understand Patient Experience Journey to Improve Pharma Value Chain
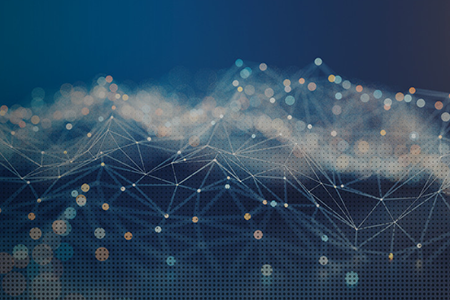
Automated Classification and Entity Extraction from essential documents pertaining to Clinical Trials
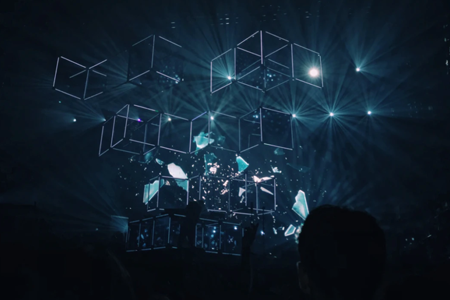
Accelerating Biomedical Innovation by Combining NLP and Knowledge Graphs
Biomedical NLP in Action
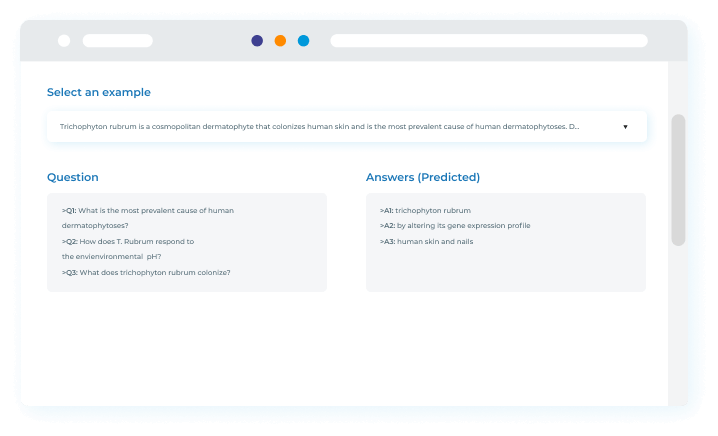
answering
biomedical research
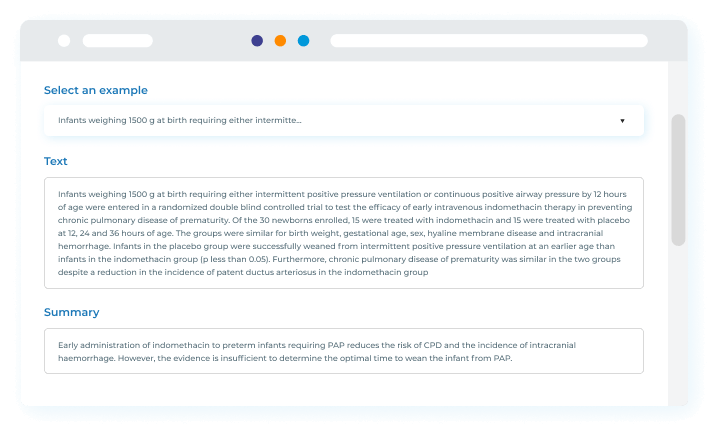
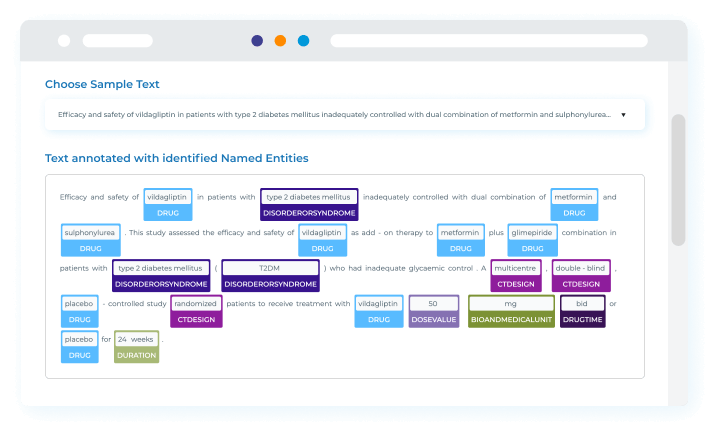
Trial Protocols
Drug Events
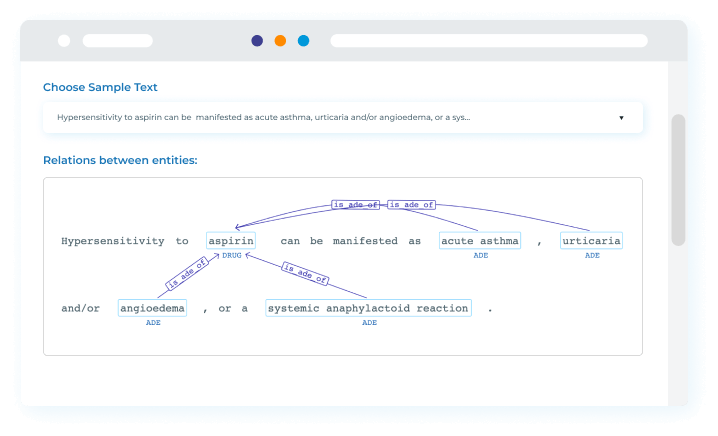
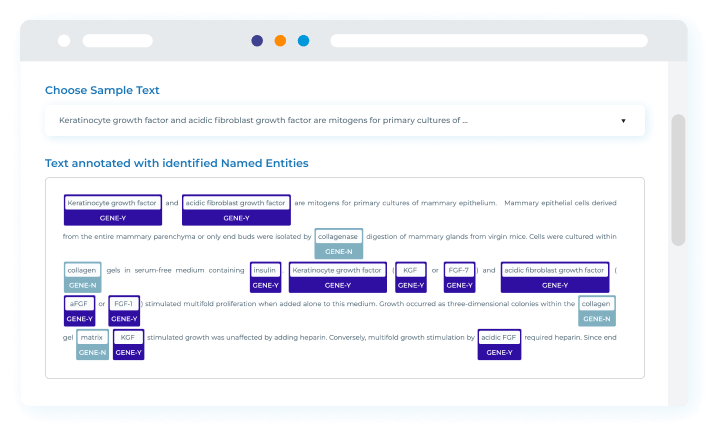
recognition
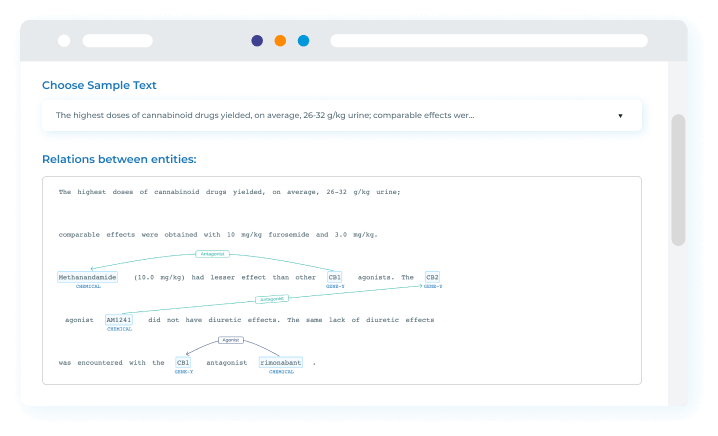
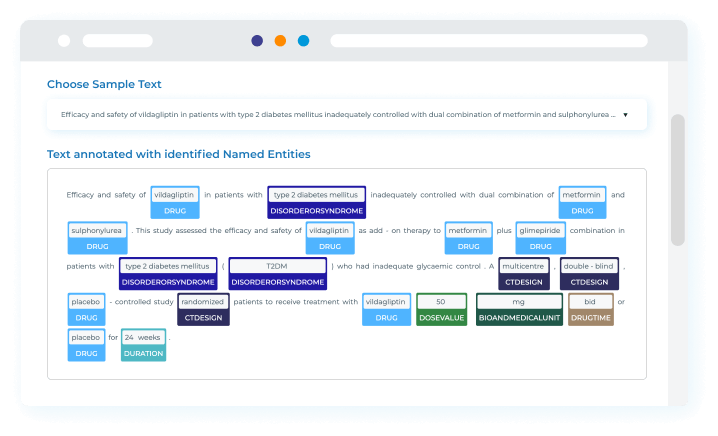
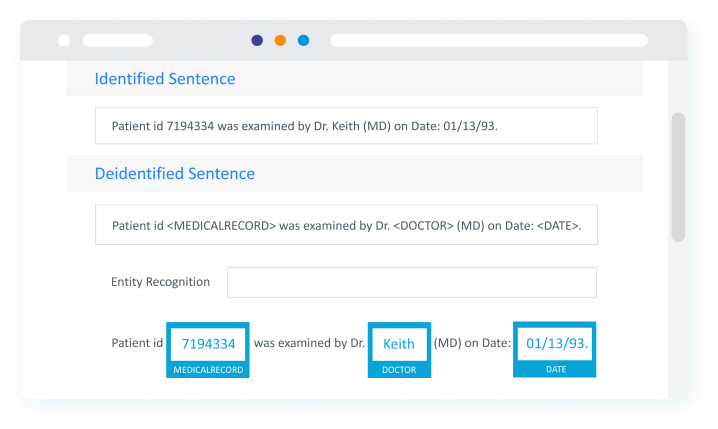
For Data Scientists
Frequently Asked Questions
Biomedical natural language processing (NLP) refers to a specialized area that combines the strengths of language processing and biomedicine intricacies. This focused NLP branch centers on gleaning valuable perceptions and insights from unstructured textual data in the sphere of health and life sciences.
Utilizing sophisticated computational methods, biomedical NLP tackles the intricacies of biomedical writings, covering domains like medical literature, clinical notes, research papers, and digital health records. Through this, it unveils concealed trends, associations, and insights that support informed choices by researchers, medical practitioners, and information analysts, propelling medical advancements.
In a nutshell, biomedical NLP emerges as a catalytic element within healthcare, harbouring the potential to transform our approach in unravelling and capitalizing on extensive medical text data. By employing its applications, we open the gate to augmented research, refined clinical judgment, and deeper comprehension of the elaborate network of biomedical wisdom.
In Bioinformatics, Biomedical NLP serves as a powerful tool for:
Data Mining: NLP tools can swiftly sift through extensive biomedical literature, research articles, and clinical notes to extract relevant information. This assists in identifying genes, proteins, diseases, and their relationships, accelerating data mining processes.
Entity Recognition: With biomedical NLP, it's possible to automatically identify and categorize entities like genes, proteins, and diseases in texts. This speeds up the creation of structured databases for further analysis.
Knowledge Discovery: NLP techniques help uncover hidden associations and patterns within biomedical data, leading to the discovery of novel insights, potential biomarkers, and therapeutic targets.
Biological Network Analysis: By analyzing biomedical text, NLP can assist in building intricate biological networks that map out interactions between genes, proteins, and molecular pathways, providing a comprehensive view of biological processes.
Text Summarization: Biomedical NLP tools facilitate the summarization of complex research articles, aiding researchers in quickly grasping key findings and advancements in the field.
Clinical Decision Support: NLP-driven analysis of clinical notes and patient records aids healthcare professionals in making informed decisions, diagnosing diseases, and designing personalized treatment plans.
Through the lens of biomedical NLP, several critical tasks are highlighted:
Entity Recognition: Biomedical NLP systems excel in recognizing and categorizing entities such as genes, proteins, diseases, and medical concepts within unstructured texts. This task forms the foundation for organizing and classifying crucial information.
Relationship Extraction: Biomedical NLP enables the extraction of relationships and associations between various entities mentioned in biomedical texts. This capability helps unveil connections between drugs and their effects, genes and diseases, and more.
Concept Mapping: The technology aids in mapping complex biomedical concepts, creating a structured representation of the relationships between terms, entities, and ideas. This facilitates clearer visualization and understanding of intricate medical information.
Information Extraction: Biomedical NLP tools extract valuable information like drug interactions, medical procedures, and disease mentions from extensive volumes of text data, supporting the creation of comprehensive biomedical databases.
Literature Analysis: Researchers benefit from NLP-powered tools that can process and analyze vast amounts of medical literature, highlighting trends, breakthroughs, and emerging areas of interest within the biomedical field.
Clinical Insights: By analyzing clinical notes and electronic health records, biomedical NLP aids healthcare professionals in extracting actionable insights, supporting diagnosis, and guiding personalized treatment plans.
Text Summarization: NLP algorithms are capable of summarizing intricate research articles, condensing their content while retaining crucial information. This aids in quick comprehension and decision-making for researchers and practitioners.