- Start with raw multimodal clinical data
- Build a complete view of the patient over time
- Answer natural language questions
- Explain your answers and cite your sources
Automatically, privately, at scale, with state-of-the-art accuracy
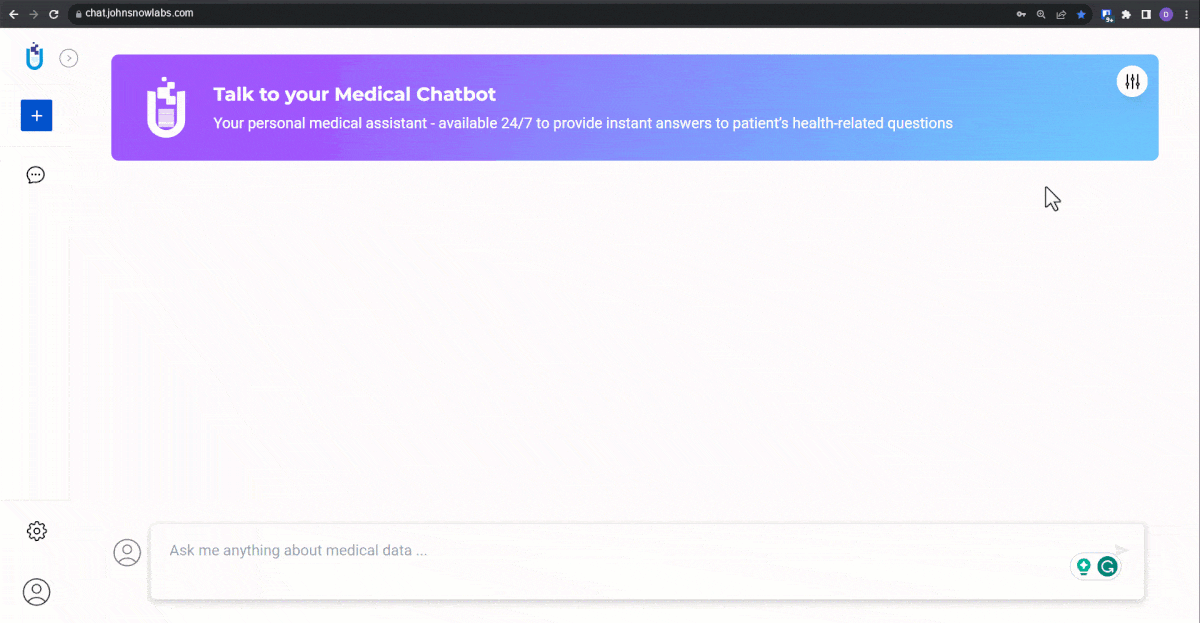
In Action: Text-Prompted Patient Cohort Retrieval
For population health managers and care management teams, segmenting high-risk patients into cohorts based on their clinical characteristics and history is desirable.
This segmentation not only allows for a better understanding of risk patterns within an individual patient, it also contextualizes these patterns across the broader patient population. Insights from the segmentation could pave the way for crafting intervention strategies tailored to address the nuances of the population.
Using John Snow Lab’s Generative Healthcare models, the ClosedLoop platform enables users to retrieve cohorts using free-text prompts. Examples include:
Which patients are in the top 5% of risk for an unplanned admission and have chronic kidney disease of stage 3 or higher?
Which patients are in the top 5% risk for an admission, older than 72, and have not undergone an annual wellness checkup?
How it Works: Patient-Level Reasoning
This is then used to either provide a user-friendly interface – such as a chatbot, search, or visual query builder – to ask questions like:
This is then used to either provide a user-friendly interface – such as a chatbot, search, or visual query builder – to ask questions like:
Has she ever been on an SSRI before?
What’s his current cancer staging?
This cannot be achieved via an LLM, RAG, NLP, or Knowledge Graph solution alone – it requires combining all of them into one end-to-end solution:
What’s his current cancer staging?
Why a Healthcare-Specific LLM: Understanding Patient Notes & Stories at the Veteran’s Administration
This case study describes benchmarks and lessons learned from building such a pilot system on data from the US Department of Veterans Affairs, a health system which serves over 9 million veterans and their families.
This collaboration with VA National Artificial Intelligence Institute (NAII), VA Innovations Unit (VAIU) and Office of Information Technology (OI&T) show that while out-of-the-box accuracy of current LLM’s on clinical notes is unacceptable, it can be significantly improved with pre-processing, for example by using John Snow Labs’ clinical text summarization models prior to feeding that as content to the LLM generative AI output.
We will also review responsible and trustworthy AI practices that are critical to delivering these technology in a safe and secure manner.
Peer-Reviewed, State-of-the-art Accuracy
“Our model achieves logical form accuracy (LFA) of 0.85 on the MIMICSQL dataset, significantly outperforming current state-of-the-art models such as Defog-SQL-Coder, GPT-3.5-Turbo, LLaMA-2-7B and GPT-4.
This approach reduces the model size, lessening the amount of data and infrastructure cost required for training and serving, and improves the performance to enable the generation of much complex SQL queries.”
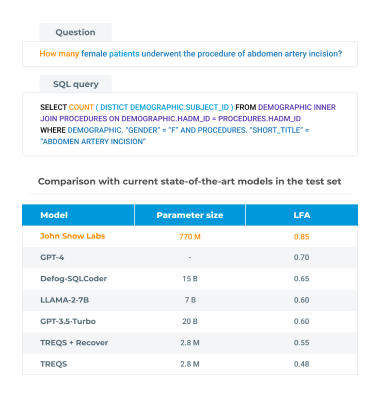