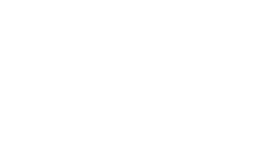
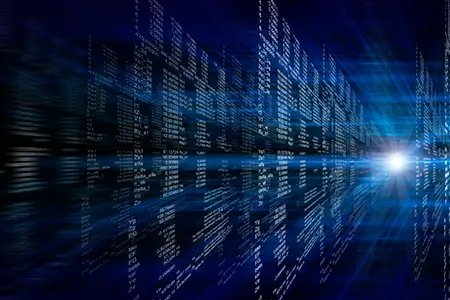
Maximizing Patient Care through AI-Enhanced HCC Code Discovery
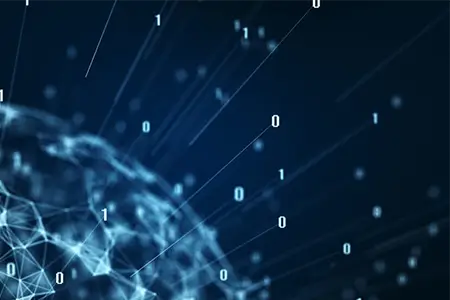
Applying Healthcare-Specific LLMs to Build Oncology Patient Timelines and Recommend Clinical Guidelines
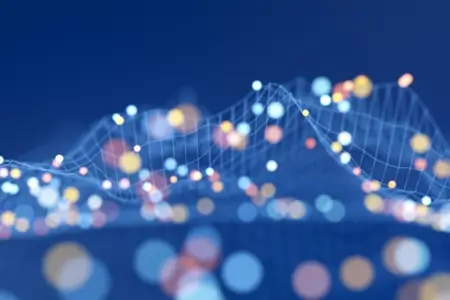
Using Generative AI for Data Extraction Clinical Support
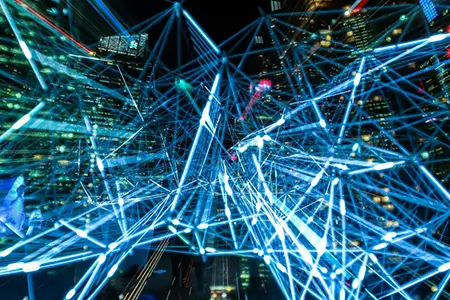
Leveraging Medical Generative AI & Agents to Help Clinicians Provide Evidence-Based Care
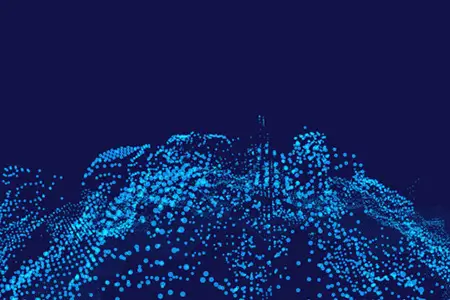
AI-Enhanced Oncology Data: Unlocking Insights from EHRs with NLP and LLMs
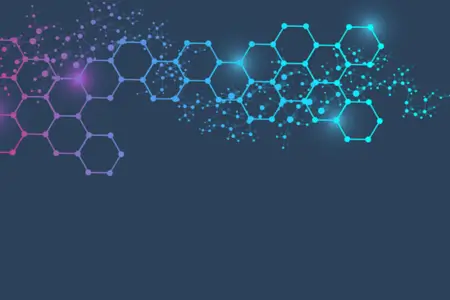
Identifying opioid-related adverse events from unstructured text in electronic health records
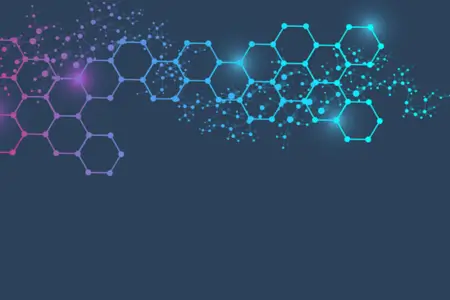
Empowering Healthcare through NLP: Harnessing Clinical Document Insights at Intermountain Health
Deidentifying Free-Text Patient Notes: No Need for Tradeoffs
Using Robotics and Generative AI Medical Language Models at the Point of Care
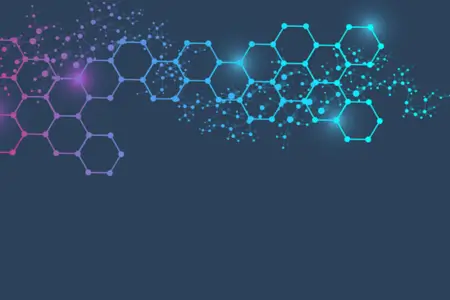
Matching Patients and Answers to the Largest Clinical Guidelines Library in the World
Productizing Healthcare ChatBots with John Snow Labs’ Medical LLM-as-a-Judge
RAG on FHIR: Using FHIR with Generative AI to Make Healthcare Less Opaque
Extracting what, when, why, and how from Radiology Reports in Real World Data acquisition projects
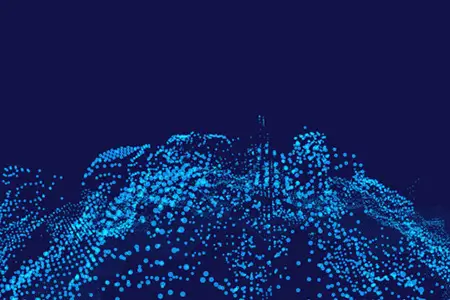
Lessons Learned De-Identifying 700 Million Patient Notes with Spark NLP
Using Healthcare-Specific LLM’s for Data Discovery from Patient Notes & Stories
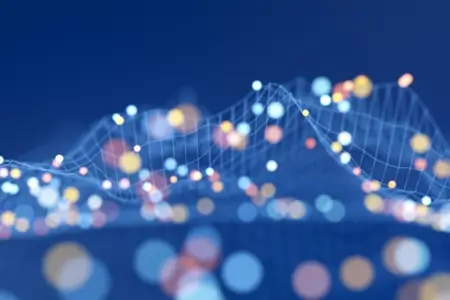
NLP for Finance – Automated Invoice Classification for Submission Compliance
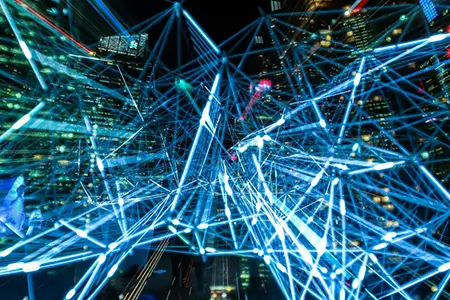
Identifying mental health concerns, subtypes, temporal patterns, and differential risks among children with Cerebral Palsy using NLP on EHR data
Leveraging Healthcare NLP Models in Regulatory Grade Oncology Data Curation
Large Language Models to Facilitate Building of Cancer Data Registries
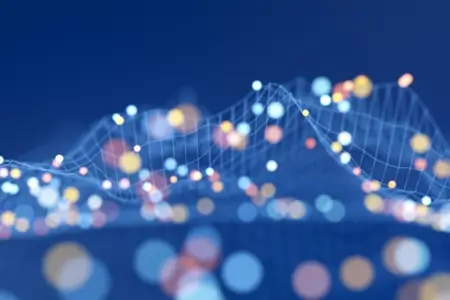
Automated Extraction of Medical Risk Factors for Life Insurance Underwriting
Automated Classification and Entity Extraction from essential documents pertaining to Clinical Trials
Therapy Specific Outcomes – Rheumatology Insights using NLP
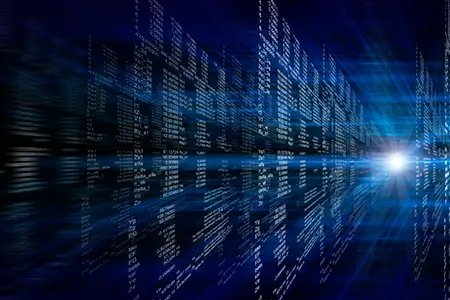
Building an Integrated Data Approach to Pharma Medical Affairs
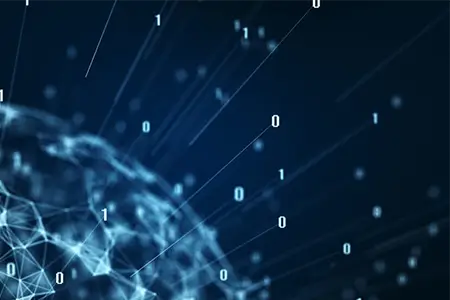
Artificial Intelligence for Pharmacovigilance Processing
John Snow Labs’ Spark NLP for Healthcare Library Speeds Up Automated Language Processing with Intel® AI Technologies
Understand Patient Experience Journey to Improve Pharma Value Chain
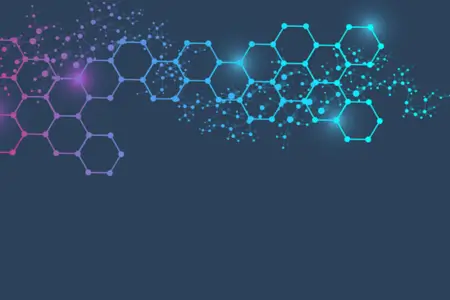
Building Reproducible Evaluation Processes for Spark NLP Models
How Care-Connect and Spryfox use NLP in Making Patient-level Decisions
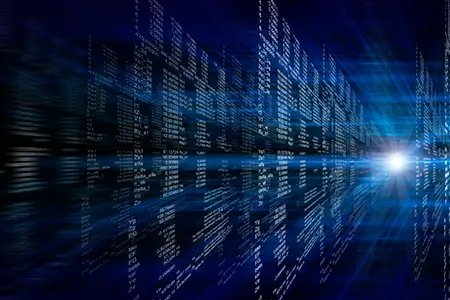
Building Patient Cohorts with NLP and Knowledge Graphs
Vakilsearch Understands Scanned Legal & Tax Forms Using John Snow Labs
Detecting Undiagnosed Conditions and Automating Medicare Risk Adjustment
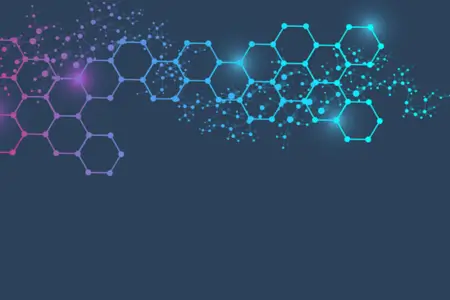
A unified CV, OCR, and NLP approach for scalable document understanding at DocuSign
Adverse Drug Event Detection Using Spark NLP
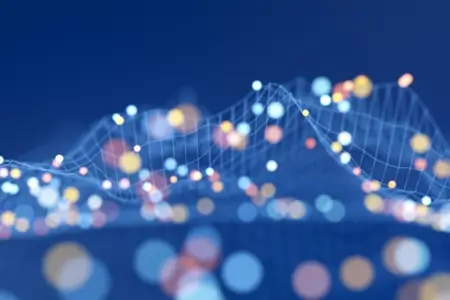
Harnessing Causality, Encoded Clinical Knowledge, and Transparency: How Ronin Enables Personalized Decisions for Cancer Patients
Databricks Introduces Partner Connect with Seamless John Snow Labs Integration
Automated Patient Risk Adjustment and Medicare HCC Coding from Clinical Notes
Using Spark NLP in R: a Drug Standardization Case Study
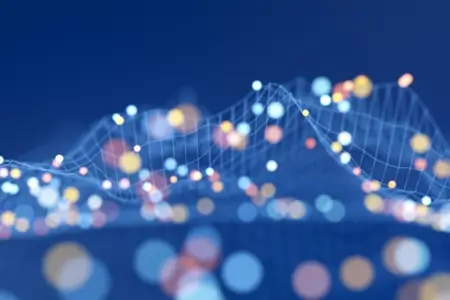
SelectData interprets millions of patient stories with deep learned OCR and NLP
Spark NLP in action: intelligent, high-accuracy fact extraction from long financial documents
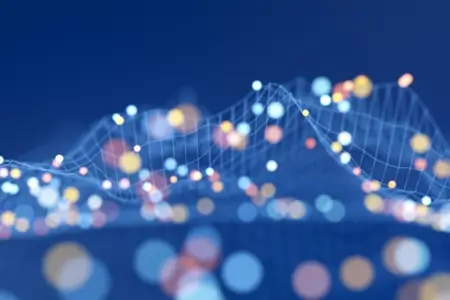
Using Spark NLP to De-Identify Doctor Notes in the German Language
Identifying Housing Insecurity and Other Social Determinants of Health from Free-Text Notes
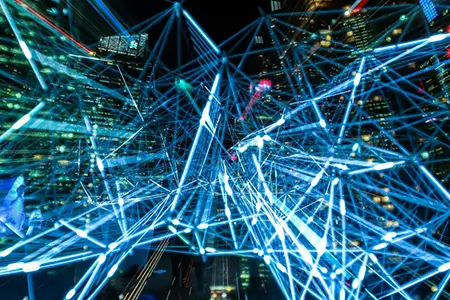
Accelerating clinical risk adjustment through Natural Language Processing
Deep6 accelerates clinical trial recruitment with Spark NLP
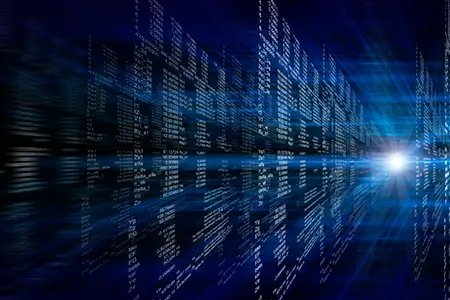
Text Classification into a Hierarchical Market Taxonomy using Spark NLP at Bitvore
ESG Document Classification
Applying advanced analytics to help improve mental health among HIV positive adolescents in South Africa
SelectData uses AI to better understand home health patients
Best Practices in Improving NLP Accuracy for Clinical Entity Recognition & Resolution
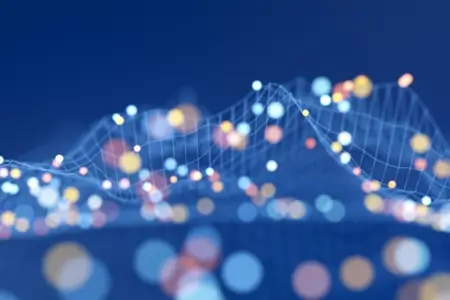
Using Spark NLP to Enable Real-World Evidence (RWE) and Clinical Decision Support in Oncology
Relation Extraction from Pathology, Radiology, and Genomic Sequencing Reports
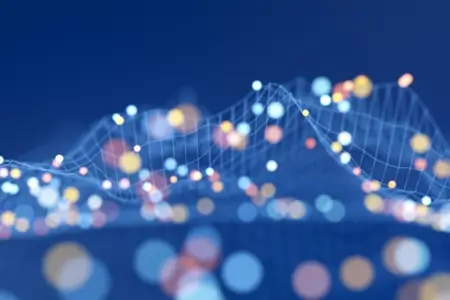
Automating a Streaming Pipeline with OCR on Databricks Lakehouse
Beyond Context: Answering Deeper Questions by Combining Spark NLP and Graph Database Analytics
Abstracting Real World Data from Oncology Notes
Benefits and Challenges in de-identifying and linking unstructured medical records
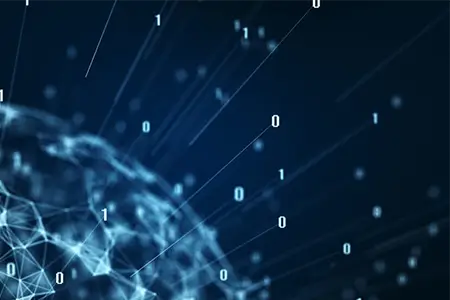
Predictive Maintenance by Intelligent Mining of Manufacturing Incidents Using Spark NLP
Accelerating Biomedical Innovation by Combining NLP and Knowledge Graphs
Sentiment Analysis of Insurance Claims Using Spark NLP
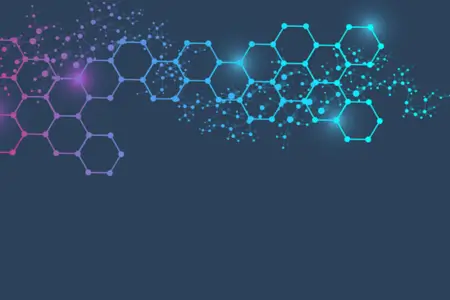
Text-Prompted Cohort Retrieval: Leveraging Generative Healthcare Models for Precision Population Health Management
Automated question answering about clinical guidelines
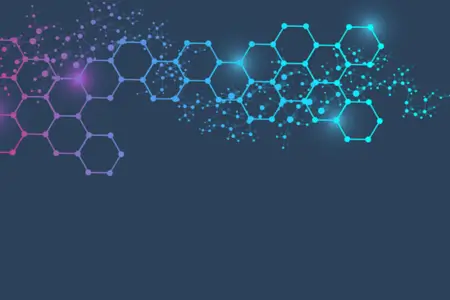
Applying State-of-the-art Natural Language Processing for Personalized Healthcare
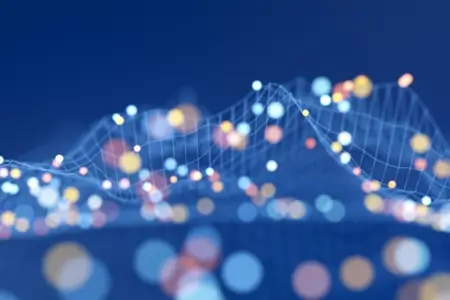
Spark NLP in Action: Improving Patient Flow Forecasting at Kaiser Permanente
Using NLP to Improve Radiologist Productivity & Reduce Burnout
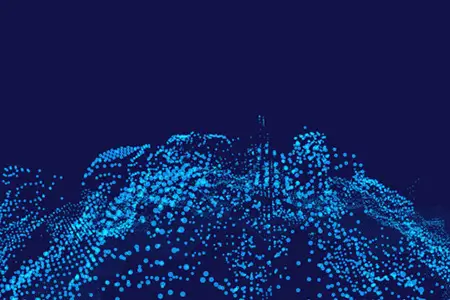
Building a Better Patient Chart: Combining structured, unstructured, and missing data
Automated Detection of Environmental, Social, and Governance Issues in Financial Documents
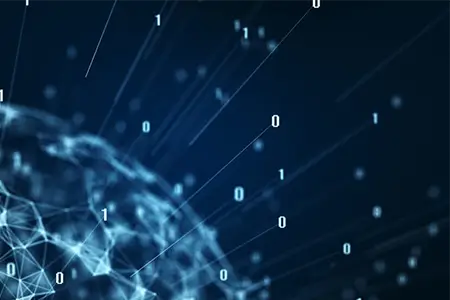
Automated and Explainable Deep Learning for Clinical Language Understanding at Roche
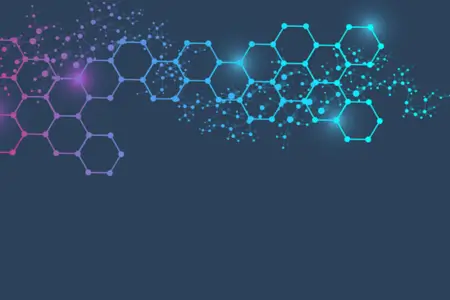
Automating PHI Removal from Healthcare Data With Natural Language Processing
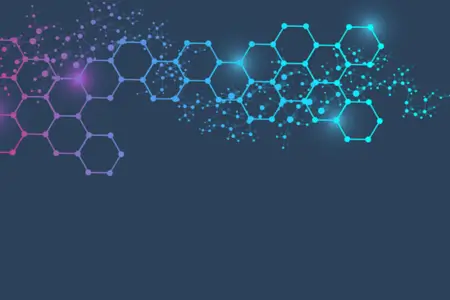
Improving Drug Safety With Adverse Event Detection Using NLP
Building, Analyzing and Querying Biomedical Knowledge Graphs using Graphster
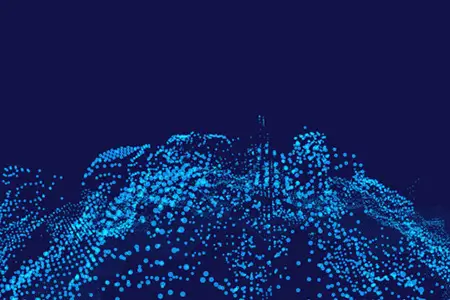
Unexpected Issues in Pharmacoepidemiology Studies Applying Natural Language Processing to Clinical Notes
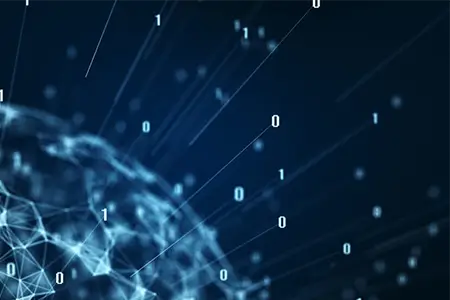
Roche automates knowledge extraction from pathology reports
Building a Smart Safety Data Sheet Parser Using NLP Lab
Identifying Patterns of Racial Discrimination through Natural Language Processing
Developing Guidelines for Responsible Generative AI in Healthcare
Adapting LLM & NLP Models to Domain-Specific Data 10x Faster with Better Data
CONAN: A Semantic Search System for Contract Analytics
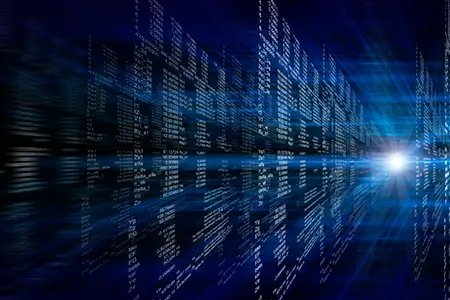
Using NLP to Identity the Emotional State of Patients at Different Stages of a Disease Journey
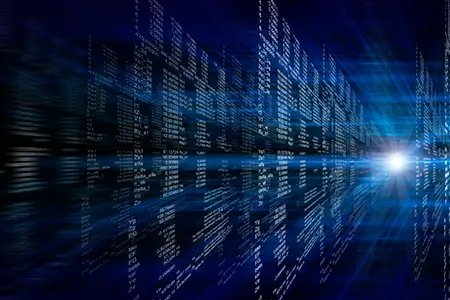
Using Real-World Data to Better Understand Inflammatory Bowel Disease (IBD)
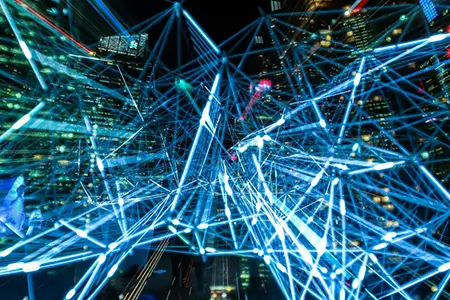