Radiology plays a critical role in healthcare as it enables clinicians to diagnose and treat various medical conditions using medical imaging techniques. These techniques include Computed Tomography (CT), X-rays, Magnetic Resonance Imaging (MRI), and Ultrasound, etc.
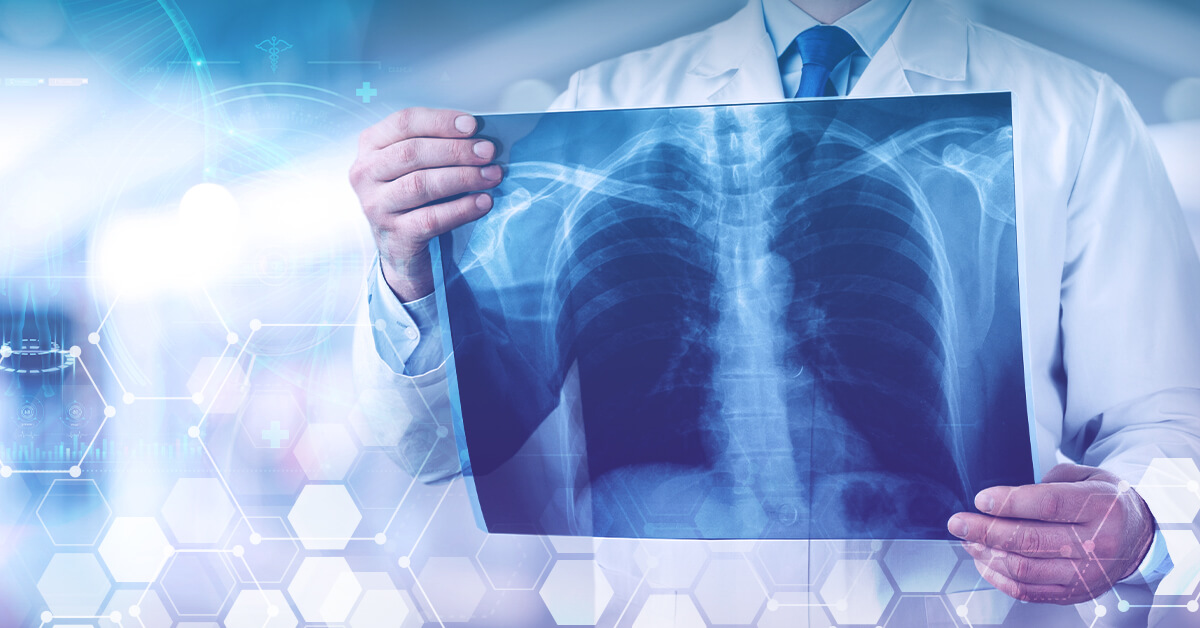
Radiology is particularly essential in the diagnosis and treatment of cancer. CT scans and MRI detect and stage cancer, monitor treatment response, and detect cancer recurrence. The interventional radiology techniques such as ablation and biopsy can be used to diagnose and treat cancer without invasive surgery. So, the key benefit of radiology is that it provides painless, non-invasive, and safe diagnostic testing. It allows doctors to timely identify potential issues and diagnose medical conditions without the need for surgeries. Moreover, radiology plays a crucial role in emergency medicine where it rapidly diagnoses traumatic injuries, strokes, and pulmonary embolism (PE). It is also essential in paediatrics enabling clinicians to diagnose and treat children’s conditions without exposing them to potentially harmful radiations.
Natural Language Processing can make radiology even more effective in diagnosing and treating various medical conditions. Let’s see how NLP in radiology can streamline workflows along with some practical examples.
Notable Applications of NLP in Radiology
In Radiology, Medical Natural Language Processing can be used in various ways to improve patient outcomes and enhance the overall quality of care. Below are the notable examples that depict how we can harness the power of NLP solutions for healthcare in radiology.
- NLP for Report Generation – Radiologists generate reports containing diagnostic information such as the presence of tumors, fractures, and other abnormalities. But these reports are complex and lengthy, making it challenging to extract relevant information. Natural language processing in radiology can help parse these reports and extract relevant information using various techniques like Named Entity Recognition (NER), Relation Extraction (RE), and Text classification.
- Named Entity Recognition identifies and extracts important entities from radiology reports such as body parts, symptoms and diseases. For instance, using NER, a system can identify and extract entities like “mass”, “liver”, and “metastasis” from radiology reports.
- Relation extraction identifies the relationships between entities. For instance, using RE, a system can identify that “mass” and “metastasis” are related to the entity “liver”, indicating the presence of liver metastases.
- Text classification identifies the overall findings of radiology reports, such as the presence and absence of tumors/abnormalities. It is particularly useful in situations where a radiologist’s interpretation may be ambiguous or difficult to parse (such as when dealing with complex imaging studies).
- NLP for Clinical Decision Support – One of the biggest challenges faced by radiologists is the sheer amount of data contained in radiology reports. These reports are quite lengthy and are difficult for a human to parse through in an accurate and timely manner. NLP clinical algorithms can be trained to identify key phrases such as “mass present” or “abnormally detected” indicating a potentially serious condition. This way healthcare providers can quickly identify areas of concern and prioritize their attention accordingly. NLP can also identify patterns in radiology reports that may be missed by human readers. For instance, it can detect a trend of increasing or decreasing tumor size over time, helping providers make more informed decisions about the most appropriate course of content. Further, NLP in radiology can flag reports that contain conflicting diagnosis or contradictory information, and can prompt a second look from a radiologist/healthcare provider.
- NLP for Image Analysis – NLP in radiology automates image analysis by extracting meaningful information from radiology images such as the size of a tumor or the presence of a particular type of tissue. This is typically achieved via a technique called “Image Captioning” in which an NLP model is trained to generate an image’s textual description. For instance, in the context of radiology, an NLP model can analyze a chest X-ray and detect the presence of lung nodules or other abnormalities. It can generate a textual description of the shape, size, and location of these nodules (along with other relevant features such as the presence of calcifications) and indicators of malignancy. Radiologists can use this information to make more informed decisions about patient care and determine if further diagnostic testing or treatment is necessary.
- NLP for Patient Monitoring – Natural language processing in radiology helps providers monitor patients over time by analyzing radiology reports in various ways. For instance, by analyzing a series of radiology images, NLP algorithms can provide quantitative data on tumor growth or regression that can be used to adjust treatment plans and assess patient progress. They can also extract information on comorbidities and other medical conditions from radiology reports, and provide a more complete picture of a patient’s health status.
- NLP for Automated Clinical Entity Detection in Radiology reports – NLP in radiology automatically identifies entities such as body parts, imaging tests, imaging results and diseases in reports. By automatically identifying clinical entities, NLP solutions for healthcare equips radiologists with a comprehensive understanding of the findings within radiology reports. Instead of manually scanning through lengthy reports, NLP swiftly identifies and extracts relevant information, presenting it in a structured and organized manner.
- NLP for Detection of anatomical and observation entities in chest radiology reports – Anatomical entities refer to specific structures/parts within the chest such as the heart, lungs, and ribs. On the other hand, observation entities refer to findings or abnormalities in the chest radiology images such as masses, nodules, or pleural effusions. NLP algorithms analyze the text of a radiology report, and look for patterns/keywords that indicate the presence of these entities (lung, heart, rib, mass, nodule, etc). Once the entities are identified, NLP extracts relevant information such as the location, size, and characteristics of abnormalities in a structured format. The demo shows how medical NLP extracts anatomical and observation entities from chest radiology reports.
- NLP for assigning an assertion status (confirmed, suspected or negative) to image findings – NLP in radiology assigns an assertion status to image findings and determines the level of certainty or confidence associated with the statement. For instance, if a report says, “There is a 5 cm mass in the left lung consistent with malignancy,” NLP recognizes the finding “ 5 cm mass” as a relevant entity and assigns the assertion status “confirmed” to it. However, if the report states: “There is a small opacity in the right lung, suspicious for malignancy,” NLP recognizes the finding “small opacity” as a relevant entity and assigns the assertion status “suspected” to it, as the language used suggests a level of certainty. The demo shows how NLP in Radiology detects imaging-findings in reports as confirmed, suspected or negative.
- NLP for Identification of relations between problems, tests and findings – If a radiology report mentions “mass” in the lungs and a subsequent biopsy, NLP identifies the relationship between the problem (lung mass), the test (biopsy), and the finding ( positive or negative for cancer). This way the healthcare providers quickly understand the clinical context of a report and make more informed decisions about patient care. The demo shows how NLP identifies relations between problems, tests and findings in radiology reports.
NLP in Radiology: In a Nutshell
Radiology plays a crucial role in healthcare as it enables physicians to visualize internal structures and diagnose various medical conditions. However, the amount of data in the radiology reports can be overwhelming, and it can be challenging for healthcare providers to extract relevant information quickly and accurately. This is where NLP comes in. It parses radiology reports, analyzes the vast amount of data, automates image analysis, and monitors patients over time by analyzing trends in radiology reports.
NLP in Radiology also identifies clinical entities and assigns an assertion status to image findings. It plays a significant role in identifying relationships between problems, tests, and findings in radiology reports. By doing so, NLP helps radiologists make accurate diagnosis and treatment plans, leading to improved patient outcomes.
John Snow Labs’ Healthcare NLP is a suite of pre-built and customizable NLP models and pipelines designed specifically for the healthcare domain. It includes a variety of NLP tasks such as Named Entity Recognition (NER), Relation Extraction (RE), Assertion Status detection, and more, all tailored for healthcare data.
Get started here and see which Radiology-related Demo can be best applied to your use case.