The Company’s Commitment to Delivering Novel, Responsible, Production-Ready Models is Reflected by Three New Milestones in Accuracy of Medical LLMs
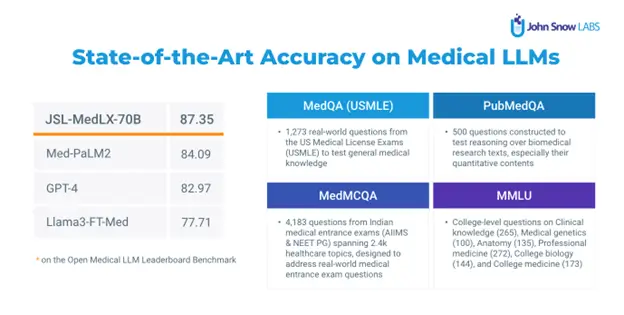
John Snow Labs, the AI for healthcare company, announced it has achieved new state-of-the-art (SOTA) medical LLM accuracy on the benchmarks used in the Open Medical LLM leaderboard, surpassing hundreds of other high performing models. This combination of nine benchmarks challenges AI models to answer thousands of medical licensing exam questions (MedQA), biomedical research questions (PubMedQA), and college-level exams in anatomy, genetics, biology, and medicine (MMLU).
The following three milestones reflect John Snow Labs’ commitment to delivering the most accurate medical Large Language Models (LLMs) to date:
- A Medical LLM which achieves 87.35 on the same reproducible test harness of the leaderboard, outperforming models such as Med-PaLM2, GPT-4, OpenBioLLMLlama, MedLlama, Orpo-Med, and all others.
- A Medical LLM with just 7 billion parameters which outperforms all previous models of that size and is the first 7B model to outperform GPT-4 on PubMedQA (78.4 vs. 75.2). PubMedQA is a dataset of 273,500 questions that require reasoning over biomedical research texts, with a single human performance of 78% accuracy.
- A Medical LLM with just 3 billion parameters which outperforms all current models of that size by more than 12 points, while still being able to run on a mobile device. This is significant for medical professionals who need to process millions to billions of patient notes without straining computing budgets. This accuracy comes close to what medical LLMs with 8 billion parameters like BioMistral achieved just three months prior.
Recent research shows that lack of accuracy was the most concerning roadblock to Generative AI adoption. Despite this, a majority of GenAI projects have not yet been tested for LLM requirements. The same survey indicated a strong preference for small, task-specific language models, with 54% of respondents from large companies using healthcare-specific task-specific language models. John Snow Labs addresses the need for top accuracy and targeted models optimized for healthcare use cases.
As achievable accuracy continues to improve, so do requirements for production-ready models. To meet efficiency, scalability, compliance, and responsible AI standards, models must be updated continuously. John Snow Labs’ Healthcare NLP & LLM subscription provides access to these models for production use, while also providing continuous updates and new releases, guaranteeing customers remain state-of-the-art over time.
“It’s a great responsibility and honor to provide novel, state-of-the-art, production-ready models to the global healthcare AI community,” said David Talby, CTO, John Snow Labs. “We didn’t give these new models fancy names because we’ll have better ones next week. That’s been the essence of our work for the past seven years, and it’s what makes John Snow Labs the most comprehensive medical language understanding solution on the market.”
John Snow Labs will continue releasing new software every two weeks. Coming soon are larger models, larger context windows, new medical text summarization models (currently beating GPT-4 2:1 on blind tests by clinicians), medical speech-to-text models (for both layman and clinician speak), and medical text translation models. To learn more, visit https://www.johnsnowlabs.com/.